select nls_env
,count(*) cnt
,xmlcast(xmlagg(xmlelement(job_name, job_name||',')).extract('//text()') as clob) jobs
from dba_scheduler_jobs
group by nls_env;
Tag Archives: oracle
Laterals: is (+) documented for laterals?
I know this syntax for a long time, since when lateral() was not documented yet, but recently I found a bug: the following query successfully returns 1 row:
with a as (select level a from dual connect by level<10)
,b as (select 0 b from dual)
,c as (select 0 c from dual)
select
*
from a,
lateral(select * from b where a.a=b.b)(+) bb
--left outer join c on c.c=bb.b
where a=1;
A B
---------- ----------
1
But doesn’t if we uncomment “left join”:
with a as (select level a from dual connect by level<10)
,b as (select 0 b from dual)
,c as (select 0 c from dual)
select
*
from a,
lateral(select * from b where a.a=b.b)(+) bb
left outer join c on c.c=bb.b
where a=1;
no rows selected
And outer apply works fine:
with a as (select level a from dual connect by level<10)
,b as (select 0 b from dual)
,c as (select 0 c from dual)
select
*
from a
outer apply (select * from b where a.a=b.b) bb
left outer join c on c.c=bb.b
where a=1;
A B C
---------- ---------- ----------
1
v$sql_hint.target_level
Today I wanted to give a link to the description of v$sql_hint.target_level to show that no_parallel can be specified for statement or object, and though it’s pretty obvious, but surprisingly I haven’t found any articles or posts about it, so this short post describes it.
v$sql_hint.target_level is a bitset, where
1st bit set to 1 means that the hint can be specified on statement level,
2nd – on query block level,
3rd – on object level,
4th – on join level(for multiple objects).
Short example:
select name,sql_feature ,class,inverse ,version,version_outline ,target_level ,decode(bitand(target_level,1),0,'no','yes') Statement_level ,decode(bitand(target_level,2),0,'no','yes') Query_block_level ,decode(bitand(target_level,4),0,'no','yes') Object_level ,decode(bitand(target_level,8),0,'no','yes') Join_level from v$sql_hint h;
with hints as ( select name,sql_feature ,class,inverse ,version,version_outline ,target_level ,decode(bitand(target_level,1),0,'no','yes') Statement_level ,decode(bitand(target_level,2),0,'no','yes') Query_block_level ,decode(bitand(target_level,4),0,'no','yes') Object_level ,decode(bitand(target_level,8),0,'no','yes') Join_level from v$sql_hint h ) select * from hints where statement_level='yes' and to_number(regexp_substr(version,'^\d+')) >= 18 order by version;
Result:
NAME SQL_FEATURE CLASS VERSION TARGET_LEVEL STATEMENT_LEVEL QUERY_BLOCK_LEVEL OBJECT_LEVEL JOIN_LEVEL ----------------- --------------- -------------------- -------- ------------ --------------- ----------------- ------------ ---------- PDB_LOCAL_ONLY QKSFM_DML PDB_LOCAL_ONLY 18.1.0 1 yes no no no SUPPRESS_LOAD QKSFM_DDL SUPPRESS_LOAD 18.1.0 1 yes no no no SYSTEM_STATS QKSFM_ALL SYSTEM_STATS 18.1.0 1 yes no no no MEMOPTIMIZE_WRITE QKSFM_EXECUTION MEMOPTIMIZE_WRITE 18.1.0 1 yes no no no SKIP_PROXY QKSFM_ALL SKIP_PROXY 18.1.0 1 yes no no no CURRENT_INSTANCE QKSFM_ALL CURRENT_INSTANCE 18.1.0 1 yes no no no JSON_LENGTH QKSFM_EXECUTION JSON_LENGTH 19.1.0 1 yes no no no QUARANTINE QKSFM_EXECUTION QUARANTINE 19.1.0 1 yes no no no
Top time-consuming predicates from ASH
Sometimes it might be useful to analyze top time-consuming filter and access predicates from ASH, especially in cases when db load is spread evenly enough by different queries and top segments do not show anything interesting, except usual things like “some tables are requested more often than others”.
Of course, we can start from analysis of SYS.COL_USAGE$: col_usage.sql
[sourcecode language=”sql”]
col owner format a30
col oname format a30 heading "Object name"
col cname format a30 heading "Column name"
accept owner_mask prompt "Enter owner mask: ";
accept tab_name prompt "Enter tab_name mask: ";
accept col_name prompt "Enter col_name mask: ";
SELECT a.username as owner
,o.name as oname
,c.name as cname
,u.equality_preds as equality_preds
,u.equijoin_preds as equijoin_preds
,u.nonequijoin_preds as nonequijoin_preds
,u.range_preds as range_preds
,u.like_preds as like_preds
,u.null_preds as null_preds
,to_char(u.timestamp, ‘yyyy-mm-dd hh24:mi:ss’) when
FROM
sys.col_usage$ u
, sys.obj$ o
, sys.col$ c
, all_users a
WHERE a.user_id = o.owner#
AND u.obj# = o.obj#
AND u.obj# = c.obj#
AND u.intcol# = c.col#
AND a.username like upper(‘&owner_mask’)
AND o.name like upper(‘&tab_name’)
AND c.name like upper(‘&col_name’)
ORDER BY a.username, o.name, c.name
;
col owner clear;
col oname clear;
col cname clear;
undef tab_name col_name owner_mask;
[/sourcecode]
But it’s not enough, for example it doesn’t show predicates combinations. In this case we can use v$active_session_history and v$sql_plan:
[sourcecode language=”sql”]
with
ash as (
select
sql_id
,plan_hash_value
,table_name
,alias
,ACCESS_PREDICATES
,FILTER_PREDICATES
,count(*) cnt
from (
select
h.sql_id
,h.SQL_PLAN_HASH_VALUE plan_hash_value
,decode(p.OPERATION
,’TABLE ACCESS’,p.OBJECT_OWNER||’.’||p.OBJECT_NAME
,(select i.TABLE_OWNER||’.’||i.TABLE_NAME from dba_indexes i where i.OWNER=p.OBJECT_OWNER and i.index_name=p.OBJECT_NAME)
) table_name
,OBJECT_ALIAS ALIAS
,p.ACCESS_PREDICATES
,p.FILTER_PREDICATES
— поля, которые могут быть полезны для анализа в других разрезах:
— ,h.sql_plan_operation
— ,h.sql_plan_options
— ,decode(h.session_state,’ON CPU’,’ON CPU’,h.event) event
— ,h.current_obj#
from v$active_session_history h
,v$sql_plan p
where h.sql_opname=’SELECT’
and h.IN_SQL_EXECUTION=’Y’
and h.sql_plan_operation in (‘INDEX’,’TABLE ACCESS’)
and p.SQL_ID = h.sql_id
and p.CHILD_NUMBER = h.SQL_CHILD_NUMBER
and p.ID = h.SQL_PLAN_LINE_ID
— если захотим за последние 3 часа:
— and h.sample_time >= systimestamp – interval ‘3’ hour
)
— если захотим анализируем предикаты только одной таблицы:
— where table_name=’&OWNER.&TABNAME’
group by
sql_id
,plan_hash_value
,table_name
,alias
,ACCESS_PREDICATES
,FILTER_PREDICATES
)
,agg_by_alias as (
select
table_name
,regexp_substr(ALIAS,’^[^@]+’) ALIAS
,listagg(ACCESS_PREDICATES,’ ‘) within group(order by ACCESS_PREDICATES) ACCESS_PREDICATES
,listagg(FILTER_PREDICATES,’ ‘) within group(order by FILTER_PREDICATES) FILTER_PREDICATES
,sum(cnt) cnt
from ash
group by
sql_id
,plan_hash_value
,table_name
,alias
)
,agg as (
select
table_name
,’ALIAS’ alias
,replace(access_predicates,’"’||alias||’".’,’"ALIAS".’) access_predicates
,replace(filter_predicates,’"’||alias||’".’,’"ALIAS".’) filter_predicates
,sum(cnt) cnt
from agg_by_alias
group by
table_name
,replace(access_predicates,’"’||alias||’".’,’"ALIAS".’)
,replace(filter_predicates,’"’||alias||’".’,’"ALIAS".’)
)
,cols as (
select
table_name
,cols
,access_predicates
,filter_predicates
,sum(cnt)over(partition by table_name,cols) total_by_cols
,cnt
from agg
,xmltable(
‘string-join(for $c in /ROWSET/ROW/COL order by $c return $c,",")’
passing
xmltype(
cursor(
(select distinct
nvl(
regexp_substr(
access_predicates||’ ‘||filter_predicates
,'("’||alias||’"\.|[^.]|^)"([A-Z0-9#_$]+)"([^.]|$)’
,1
,level
,’i’,2
),’ ‘)
col
from dual
connect by
level<=regexp_count(
access_predicates||’ ‘||filter_predicates
,'("’||alias||’"\.|[^.]|^)"([A-Z0-9#_$]+)"([^.]|$)’
)
)
))
columns cols varchar2(400) path ‘.’
)(+)
order by total_by_cols desc, table_name, cnt desc
)
select
table_name
,cols
,sum(cnt)over(partition by table_name,cols) total_by_cols
,access_predicates
,filter_predicates
,cnt
from cols
where rownum<=50
order by total_by_cols desc, table_name, cnt desc;
[/sourcecode]
As you can see it shows top 50 predicates and their columns for last 3 hours. Despite the fact that ASH stores just sampled data, its results are representative enough for high-load databases.
Just few details:
- Column “COLS” shows “search columns”, and total_by_cols – their number of occurrences
- I think it’s obvious, that this info is not unambiguous marker of the problem, because for example few full table scans can misrepresent the statistics, so sometimes you will need to analyze such queries deeper (v$sqlstats,dba_hist_sqlstat)
- We need to group data by OBJECT_ALIAS within SQL_ID and plan_hash_value, because in case of index access with lookup to table(“table access by rowid”) some predicates are in the row with index access and others are in the row with table access.
Depending on the needs, we can modify this query to analyze ASH data by different dimensions, for example with additional analysis of partitioning or wait events.
Correct syntax for the table_stats hint
A friend contacted me to ask why they were having problems using the table_stats hint to influence optimizer decision making and also to influence the decision to use direct read or buffer cache scan so this is just a quick blog post to clarify the syntax as it is not well documented.
table_stats(<table_name> <method> {<keyword>=<value>} )
Method is one of: DEFAULT, SET, SCALE, SAMPLE
Keyword is one of: BLOCKS, ROWS, ROW_LENGTH
Top-N again: fetch first N rows only vs rownum
Three interesting myths about rowlimiting clause vs rownum have recently been posted on our Russian forum:
- TopN query with rownum<=N is always faster than "fetch first N rows only" (ie. row_number()over(order by ...)<=N)
- “fetch first N rows only” is always faster than rownum<=N
- “SORT ORDER BY STOPKEY” stores just N top records during sorting, while “WINDOW SORT PUSHED RANK” sorts all input and stores all records sorted in memory.
Interestingly that after Vyacheslav posted first statement as an axiom and someone posted old tests(from 2009) and few people made own tests which showed that “fetch first N rows” is about 2-3 times faster than the query with rownum, the final decision was that “fetch first” is always faster.
First of all I want to show that statement #3 is wrong and “WINDOW SORT PUSHED RANK” with row_number works similarly as “SORT ORDER BY STOPKEY”:
It’s pretty easy to show using sort trace:
Let’s create simple small table Tests1 with 1000 rows where A is in range 1-1000 (just 1 block):
create table test1(a not null, b) as select level, level from dual connect by level<=1000; alter session set max_dump_file_size=unlimited; ALTER SESSION SET EVENTS '10032 trace name context forever, level 10'; ALTER SESSION SET tracefile_identifier = 'rownum'; select * from (select * from test1 order by a) where rownum<=10; ALTER SESSION SET tracefile_identifier = 'rownumber'; select * from test1 order by a fetch first 10 rows only;
And we can see from the trace files that both queries did the same number of comparisons:
[sourcecode language=”sql” highlight=”7″]
—– Current SQL Statement for this session (sql_id=bbg66rcbt76zt) —–
select * from (select * from test1 order by a) where rownum<=10
—- Sort Statistics ——————————
Input records 1000
Output records 10
Total number of comparisons performed 999
Comparisons performed by in-memory sort 999
Total amount of memory used 2048
Uses version 1 sort
—- End of Sort Statistics ———————–
[/sourcecode]
[sourcecode language=”sql” highlight=”7″]
—– Current SQL Statement for this session (sql_id=duuy4bvaz3d0q) —–
select * from test1 order by a fetch first 10 rows only
—- Sort Statistics ——————————
Input records 1000
Output records 10
Total number of comparisons performed 999
Comparisons performed by in-memory sort 999
Total amount of memory used 2048
Uses version 1 sort
—- End of Sort Statistics ———————–
[/sourcecode]
Ie. each row (except first one) was compared with the biggest value from top 10 values and since they were bigger than top 10 value, oracle doesn’t compare it with other TopN values.
And if we change the order of rows in the table both of these queries will do the same number of comparisons again:
[sourcecode language=”sql”]
create table test1(a not null, b) as
select 1000-level, level from dual connect by level<=1000;
alter session set max_dump_file_size=unlimited;
ALTER SESSION SET EVENTS ‘10032 trace name context forever, level 10’;
ALTER SESSION SET tracefile_identifier = ‘rownum’;
select * from (select * from test1 order by a) where rownum<=10;
ALTER SESSION SET tracefile_identifier = ‘rownumber’;
select * from test1 order by a fetch first 10 rows only;
[/sourcecode]
[sourcecode language=”sql” highlight=”7″]
—– Current SQL Statement for this session (sql_id=bbg66rcbt76zt) —–
select * from (select * from test1 order by a) where rownum<=10
—- Sort Statistics ——————————
Input records 1000
Output records 1000
Total number of comparisons performed 4976
Comparisons performed by in-memory sort 4976
Total amount of memory used 2048
Uses version 1 sort
—- End of Sort Statistics ———————–
[/sourcecode]
[sourcecode language=”sql” highlight=”7″]
—– Current SQL Statement for this session (sql_id=duuy4bvaz3d0q) —–
select * from test1 order by a fetch first 10 rows only
—- Sort Statistics ——————————
Input records 1000
Output records 1000
Total number of comparisons performed 4976
Comparisons performed by in-memory sort 4976
Total amount of memory used 2048
Uses version 1 sort
—- End of Sort Statistics ———————–
[/sourcecode]
We can see that both queries required much more comparisons(4976) here, that’s because each new value is smaller than the biggest value from the topN and even smaller than lowest value, so oracle should get right position for it and it requires 5 comparisons for that (it compares with 10th value, then with 6th, 3rd, 2nd and 1st values from top10). Obviously it makes less comparisons for the first 10 rows.
Now let’s talk about statements #1 and #2:
We know that rownum forces optimizer_mode to switch to “first K rows”, because of the parameter “_optimizer_rownum_pred_based_fkr”
SQL> @param_ rownum NAME VALUE DEFLT TYPE DESCRIPTION ---------------------------------- ------ ------ --------- ------------------------------------------------------ _optimizer_rownum_bind_default 10 TRUE number Default value to use for rownum bind _optimizer_rownum_pred_based_fkr TRUE TRUE boolean enable the use of first K rows due to rownum predicate _px_rownum_pd TRUE TRUE boolean turn off/on parallel rownum pushdown optimization
while fetch first/row_number doesn’t (it will be changed after the patch #22174392) and it leads to the following consequences:
1. first_rows disables serial direct reads optimization(or smartscan on Exadata), that’s why the tests with big tables showed that “fetch first” were much faster than the query with rownum.
So if we set “_serial_direct_read”=always, we get the same performance in both tests (within the margin of error).
2. In cases when index access (index full scan/index range scan) is better, CBO differently calculates the cardinality of underlying INDEX FULL(range) SCAN:
the query with rownum is optimized for first_k_rows and the cardinality of index access is equal to K rows, but CBO doesn’t reduce cardinality for “fetch first”, so the cost of index access is much higher, compare them:
[sourcecode language=”sql” highlight=”13″]
SQL> explain plan for
2 select *
3 from (select * from test order by a,b)
4 where rownum<=10;
——————————————————————————————–
| Id | Operation | Name | Rows | Bytes | Cost (%CPU)| Time |
——————————————————————————————–
| 0 | SELECT STATEMENT | | 10 | 390 | 4 (0)| 00:00:01 |
|* 1 | COUNT STOPKEY | | | | | |
| 2 | VIEW | | 10 | 390 | 4 (0)| 00:00:01 |
| 3 | TABLE ACCESS BY INDEX ROWID| TEST | 1000K| 12M| 4 (0)| 00:00:01 |
| 4 | INDEX FULL SCAN | IX_TEST_AB | 10 | | 3 (0)| 00:00:01 |
——————————————————————————————–
Predicate Information (identified by operation id):
—————————————————
1 – filter(ROWNUM<=10)
[/sourcecode]
[sourcecode language=”sql” highlight=”13″]
SQL> explain plan for
2 select *
3 from test
4 order by a,b
5 fetch first 10 rows only;
—————————————————————————————–
| Id | Operation | Name | Rows | Bytes |TempSpc| Cost (%CPU)| Time |
—————————————————————————————–
| 0 | SELECT STATEMENT | | 10 | 780 | | 5438 (1)| 00:00:01 |
|* 1 | VIEW | | 10 | 780 | | 5438 (1)| 00:00:01 |
|* 2 | WINDOW SORT PUSHED RANK| | 1000K| 12M| 22M| 5438 (1)| 00:00:01 |
| 3 | TABLE ACCESS FULL | TEST | 1000K| 12M| | 690 (1)| 00:00:01 |
—————————————————————————————–
Predicate Information (identified by operation id):
—————————————————
1 – filter("from$_subquery$_002"."rowlimit_$$_rownumber"<=10)
2 – filter(ROW_NUMBER() OVER ( ORDER BY "TEST"."A","TEST"."B")<=10)
[/sourcecode]
[sourcecode language=”sql” highlight=”14″]
SQL> explain plan for
2 select/*+ first_rows */ *
3 from test
4 order by a,b
5 fetch first 10 rows only;
——————————————————————————————–
| Id | Operation | Name | Rows | Bytes | Cost (%CPU)| Time |
——————————————————————————————–
| 0 | SELECT STATEMENT | | 10 | 780 | 27376 (1)| 00:00:02 |
|* 1 | VIEW | | 10 | 780 | 27376 (1)| 00:00:02 |
|* 2 | WINDOW NOSORT STOPKEY | | 1000K| 12M| 27376 (1)| 00:00:02 |
| 3 | TABLE ACCESS BY INDEX ROWID| TEST | 1000K| 12M| 27376 (1)| 00:00:02 |
| 4 | INDEX FULL SCAN | IX_TEST_AB | 1000K| | 2637 (1)| 00:00:01 |
——————————————————————————————–
Predicate Information (identified by operation id):
—————————————————
1 – filter("from$_subquery$_002"."rowlimit_$$_rownumber"<=10)
2 – filter(ROW_NUMBER() OVER ( ORDER BY "TEST"."A","TEST"."B")<=10)
[/sourcecode]
[sourcecode language=”sql” highlight=”14″]
SQL> explain plan for
2 select/*+ index(test (a,b)) */ *
3 from test
4 order by a,b
5 fetch first 10 rows only;
——————————————————————————————–
| Id | Operation | Name | Rows | Bytes | Cost (%CPU)| Time |
——————————————————————————————–
| 0 | SELECT STATEMENT | | 10 | 780 | 27376 (1)| 00:00:02 |
|* 1 | VIEW | | 10 | 780 | 27376 (1)| 00:00:02 |
|* 2 | WINDOW NOSORT STOPKEY | | 1000K| 12M| 27376 (1)| 00:00:02 |
| 3 | TABLE ACCESS BY INDEX ROWID| TEST | 1000K| 12M| 27376 (1)| 00:00:02 |
| 4 | INDEX FULL SCAN | IX_TEST_AB | 1000K| | 2637 (1)| 00:00:01 |
——————————————————————————————–
Predicate Information (identified by operation id):
—————————————————
1 – filter("from$_subquery$_002"."rowlimit_$$_rownumber"<=10)
2 – filter(ROW_NUMBER() OVER ( ORDER BY "TEST"."A","TEST"."B")<=10)
[/sourcecode]
So in this case we can add hints “first_rows” or “index”, or install the patch #22174392.
ps. I thought to post this note later, since I hadn’t time enough to add other interesting details about the different TopN variants, including “with tie”, rank(), etc, so I’ll post another note with more details later.
Docker with Oracle database: install patches automatically
Recently I had to install the patch for fixing cross-platform PDB transport bug onto the docker images with Oracle, so these are easy way how to do it:
1. create directory “patches” and create “install_patches.sh”:
#!/bin/bash unzip -u ./*.zip CURDIR=`pwd` for D in *; do if [ -d "${D}" ]; then echo ================================================= echo " *** Processing patch # ${D}... " # your processing here cd "${D}" opatch apply -silent fi cd $CURDIR done
2. add the following commands into the dockerfile:
USER root # Copy DB install file COPY --chown=oracle:dba patches $INSTALL_DIR/patches # Install DB software binaries USER oracle RUN chmod ug+x $INSTALL_DIR/patches/*.sh && \ sync && \ cd $INSTALL_DIR/patches && \ ./install_patches.sh
3. put downloaded patches into the “patches” directory and build image.
For example, dockerfile for 18.3:
FROM oracle/database:18.3.0-ee MAINTAINER Sayan Malakshinov <sayan@orasql.org> USER root # Copy patches: COPY --chown=oracle:dba patches $INSTALL_DIR/patches # Install patches: USER oracle RUN chmod ug+x $INSTALL_DIR/patches/*.sh && \ sync && \ cd $INSTALL_DIR/patches && \ ./install_patches.sh
ps. I’ve also create the request for that in the official Docker github: https://github.com/oracle/docker-images/issues/1070
Compression in a well-balanced system
Exadata is designed to present a well-balanced system between disk scan rates, cell CPU processing rates, network bandwidth, and RDBMS CPU such that on average across a lot of workloads and a lot of applications there should not be no one obvious bottleneck. Good performance engineering means avoiding a design like this:
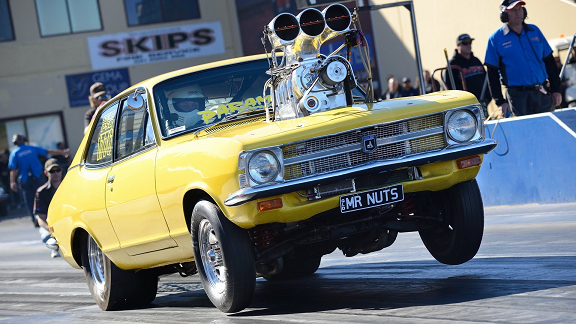
because that would be nuts. If you change one component in a car e.g. the engine you need to rework the other components such as the chassis and the brakes. When we rev the Exadata hardware we go through the same exercise to make sure the components match to avoid bottlenecks.
One of the components in a well-balanced system that people often leave off this list is compression algorithm. With compression you are balancing storage costs as well as the disk scan rates against the CPU’s decompression rate. In a disk bound system you can alleviate the bottleneck by compressing the data down to the point that it is being scanned at the same rate that the CPUs can decompress and process it. Squeeze it too far, say with HCC “Compress For Archive High” which uses BZ2, the CPUs become a major bottleneck because the decompression costs unbalance the system (but it’s wonderful if you think you’re unlikely to ever need the data again).
Continue readingCreate Quarantine
First if you want don’t know what an Exadata Quarantine is read this.
Someone asked whether you can create your own Exadata Cell quarantine and, if you can, why you might ever want to do it?
The first step when you don’t know how to do something is try HELP in cellcli
CellCLI> HELP
...
ALTER QUARANTINE
...
CREATE QUARANTINE
...
DROP QUARANTINE
...
LIST QUARANTINE
So we see we can create a quarantine, so we use HELP again:
Continue readingShining some light on Database In-Memory vs the Exadata Columnar Cache in 12.1.0.2
I posted a while back on how to use Tracing Hybrid Columnar Compression in an offload server so this is a quick follow up.
- I have trouble remembering the syntax for setting a regular parameter in an offload server without bouncing it. Since I need to keep this written down somewhere I thought it might be use to support folks and dbas.
- I forgot to show you how to specify which offload group to set the trace event
So this example should do both:
CellCLI > alter cell offloadGroupEvents = "immediate cellsrv.cellsrv_setparam('my_parameter, 'TRUE')", offloadGroupName = "SYS_122110_160621"
this will, of course, set a parameter temporarily until the next time the offload server is bounced, but also adding it to the offload group’s init.ora will take care of that.